💯 An Ode to the Type A Data Scientist
Jun 28, 2018 • 7 min • Data Science
The Mythical Unicorn
You’ve probably heard of the elusive data science unicorn by now. If you haven’t, unicorns are professionals who have mastered several cross-disciplinary skills in and around the field of data science. They have all the answers to your questions on data analysis, machine learning, product metrics, big data, experimentation, deep learning, business acumen, domain knowledge and more.
A better visualization of this rare feat can be seen in the study below, polling 400+ data specialists on their range of comfortability with different areas that could be classified as those of a true unicorn.
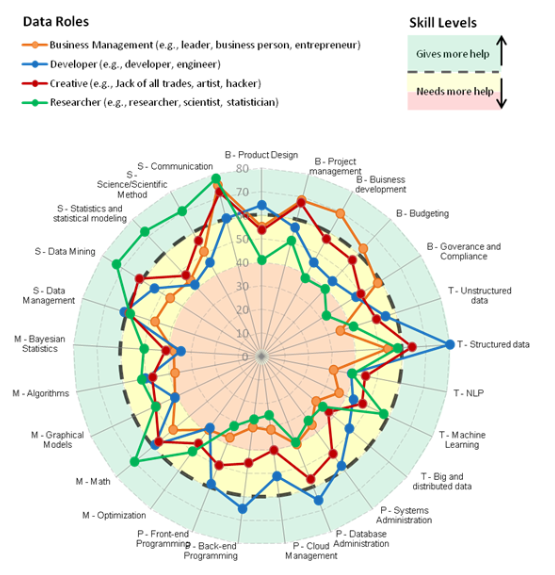
As you might imagine, finding someone that excels at all of these things certainly isn’t easy; it’s next to impossible. Due to this realization within leading companies, we’ve come to see specialized roles form within the broader field of data science.
These specializations include job titles such as Machine Learning Engineer, Data Engineer, Data Analyst, Product Scientist, Core Data Scientist, Data Researcher, Quantitative Analyst, among other roles that have grown to be common fixtures along with the more well-known Data Scientist.
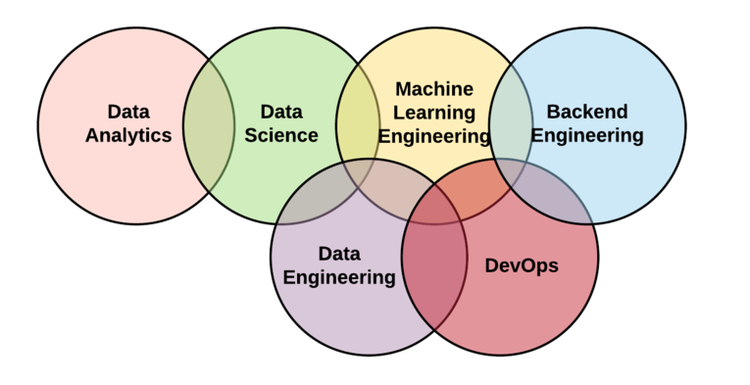
As you can see mapped out above, there will always be some sort of overlap between these roles. This will typically be specific to each company, their data ecosystem, and their future goals. Believe it or not, things don’t stop here though. We can break it down even further.
Type A vs. Type B
Many well-respected individuals in the data science community have taken a stab at classifying different types of Data Scientists. However, none more effective than Michael Hochster, PhD and former Head of Research at Pandora, with the following answer on Quora:
Type A Data Scientist: The A is for Analysis. This type is primarily concerned with making sense of data or working with it in a fairly static way. The Type A Data Scientist is very similar to a statistician (and may be one) but knows all the practical details of working with data that aren’t taught in the statistics curriculum: data cleaning, methods for dealing with very large data sets, visualization, deep knowledge of a particular domain, writing well about data, and so on.
Type B Data Scientist: The B is for Building. Type B Data Scientists share some statistical background with Type A, but they are also very strong coders and may be trained software engineers. The Type B Data Scientist is mainly interested in using data “in production.” They build models which interact with users, often serving recommendations (products, people you may know, ads, movies, search results).
These descriptions primarily apply to working with data science in industry, but I’ve found them to be spot-on in my experience. It’s also worth noting that these classifications aren’t definitive and certainly aren’t static. You may have a blend of skills that falls under both types and there’s nothing wrong with that. In fact, when done right, it can actually lead to a unique and invaluable skillset that lends itself to creative and effective solutions.
The Next Generation
If you come across data science in the news these days, whether it’s a feature in the New York Times or an article on TechCrunch, odds are that the piece is focused on the impact of machine learning and artificial intelligence.
This is for good reason. The growth of artificial intelligence and machine learning will continue to have an increasingly profound impact of all of our lives. This phenomenon affects everyone on the planet in one way or another, and vast media coverage reflects this. With this in mind, let’s explore an important question that any leader in the field should always have in the back of their mind:
What does this mean for the next generation of Data Scientists?
If you recall our in-depth classification of Data Scientist, these exciting new skills fit nicely in the Type B description focused on building and deploying models while working with data in production.
It’s clear that Type B data skills like machine learning are surrounded by more hype and appeal at the moment. For this reason, aspiring Data Scientists are entering the field with their eyes set on this particular ML-based skillset.
I mean, who doesn’t want to build machine learning models that let you predict the future? Who isn’t fascinated by recommender systems and neural networks that mimic the human brain?
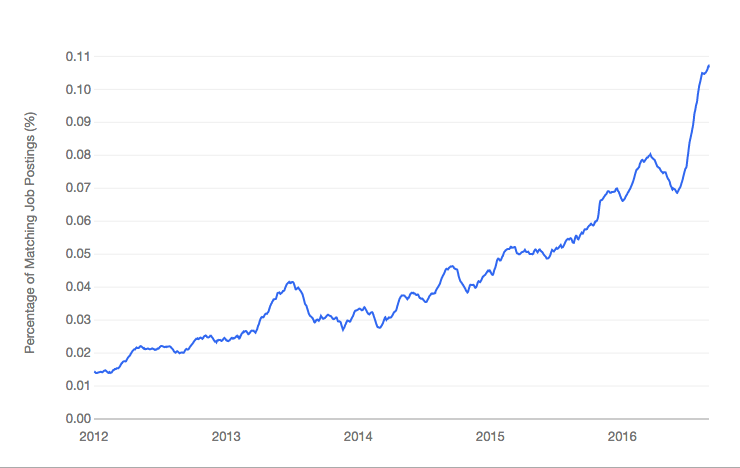
The need for these skills is certainly out there, as validated by the plethora of data-driven education initiatives and the rapidly growing pool of AI and ML Engineers at the moment.
However, this mindset is a bit shortsighted. Despite what headlines and buzzwords suggest, most problems can’t be solved with machine learning.
I would like to use the rest of this post to make the argument for the value provided by skills and actions associated with the often overlooked and under-appreciated, Type A Data Scientist.
Why should I care about Type A Data Scientists? Glad you asked…
They Answer Difficult Questions
Type A Data Scientists extract information from data in order to explore and answer complex questions typically posed by domain experts, business leaders, or management. This may not seem all that glamorous, and as often the case with data science — it isn’t. There will almost always be some sort of data wrangling and cleaning required on your part.
However, once you’re through this stage, things get interesting quickly. There is rarely a straight answer to the question posed. You have to be creative and think through a seemingly infinite selection of routes to go down.
“Critical thinking skills set apart the hackers from the true scientists” — Jake Porway
This comes off to many as a consequence of the profession. However, I beg to differ. The limitless ways to analyze and solve problems are largely what makes data science so challenging and interesting to such a large population.
They Make the Complex Simple
Arguably the most important and overlooked skill that a Data Scientist can possess is that of simple, yet thorough communication. Deconstructing complex solutions and packaging them for business leaders, product managers, or users to understand isn’t a small task.
“The numbers have no way of speaking for themselves. We speak for them. Before we demand more of our data, we need to demand more of ourselves” — Nate Silver
The ways in which we speak for numbers can vary greatly; these include presentation skills, data storytelling, data visualization, business insights, and technical writing. Being able to decide which of these tools to utilize and doing so in an effective manner isn’t easy, but it’s extremely gratifying.
They Consistently Drive Impact
Naturally, your results are only as good as the impact they drive. Type A Data Scientists will generally get the opportunity to drive more impact on a day-to-day basis than their Type B counterparts. Instead of spending time on feature engineering and iterating models, you’ll be exploring problems that directly influence decisions made for the business or product.
“No great marketing decisions have ever been made on qualitative data.” — John Sculley
The turnaround on value-adding is much faster for Type A Data Scientists as well. You’ll be getting consistent feedback on your work and because of this, you’ll have the opportunity to grow quickly as a Data Scientist.
The Hard Sell
The point of this post isn’t to discourage anyone from focusing on developing a machine learning toolkit to build powerful models. Personally, I take the time to work on developing those skills each and every day. I also believe that comfortability with data in production is an extremely useful tool to scale your projects and influence the business in unique ways.
In short, Type B data science undoubtedly has it’s upside as well. Each skillset deals with very different parts of the ecosystem. Both types live in different neighborhoods of the larger, fast-growing city of data science.
However, I believe that due to hype surrounding machine learning and artificial intelligence, the vast majority of upcoming data talent at the moment is gravitating towards Type B work. For this reason, I fear that impactful skills associated with Type A data science, primarily dealing with analysis and communication, are going overlooked.
While analysis and communication may not be as sexy as machine learning, the reality is that they offer the most consistent impact in industry.
In a world where data is the new oil, using analysis to solve problems is an extremely empowering thing. Sure, you’ll get stuck from time to time. That’s the beauty of doing hard work. There is always another challenge to tackle. Another problem to solve. Another question to answer.
For those questions, we have Type A Data Scientists.
Thanks for reading! If you enjoyed this post and you’re feeling generous, perhaps follow me on Twitter. You can also subscribe in the form below to get future posts like this one straight to your inbox. 🔥