🚀 What Should Onboarding Look Like for Data Scientists?
Apr 18, 2019 • 7 min • Data Science
Chances are, you’ve been there before. It’s no secret that onboarding is an important part of a company’s recruiting process. It consistently affects both new hires and team members, but for some reason, it’s often overlooked.
Onboarding isn’t just the first impression that a company makes; it sets the tone for the new hire’s experience there. Despite the ever-growing amount of content being churned out by the online data science community, there’s a surprisingly scarce amount of information on best practices as they relate to onboarding.
In this post, I’ll look to address this need by sharing what my experience has taught me about onboarding new hire data scientists in industry.
Data scientists need their own system
We know that data science is an inherently multidisciplinary field that requires a diverse skill set. Generalists by nature, we are expected to cover all sorts of ground between engineering, product, reporting, analysis, and more.
However, this also means that new hires need appropriate onboarding in each of these areas. This is why the task of onboarding data scientists is a bit trickier than most. You have to go broader. This means establishing a foundation of knowledge in several different areas, rather than honing in on one or two specialties.
While unavoidable, this isn’t a huge obstacle. It simply means that we need to think about onboarding a little differently for data scientists. Not a full overhaul, but we need a system that is personalized to the style of work that we will be doing.
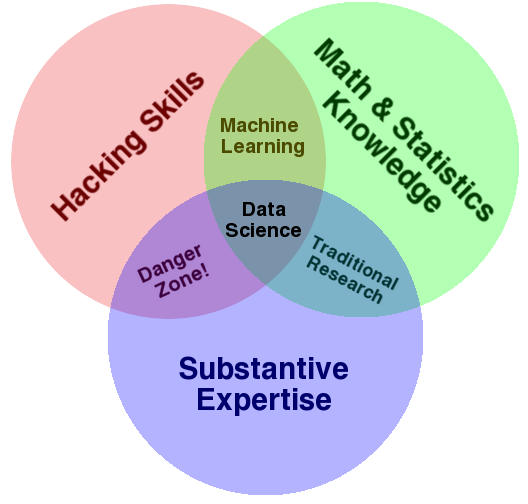
Setting expectations
Once all of the human resources stuff is out of the way and the new hire is up to speed on any company-wide information and processes, it’s time to set expectations.
This normally takes the form of an initial meeting with their manager where they can share a bunch of information and dive further into the role. The discussion should include the following before you move further into onboarding:
- Overview of the position
- Expectations that come with it
- Immediate projects to think about
- Possible projects further down the road map
- Any policies you want to establish
- Who they’ll be interacting with frequently
- Points of contact for questions
Getting to know the team
Next up, it’s time to meet the rest of the team. This step will vary per company, depending on the size of your data science team and the structure of your organization. The big thing here is making the new hire feel comfortable and getting the introductions out of the way. This can be taking a lap around the office to meet everyone or something as easy as sitting down for lunch with the team.
Once introductions are done, they should get to know everyone a bit better and start to learn more about the team. My favorite way to do this is using Andrew Bosworth of Facebook’s Career Cold Start Algorithm over the first couple of months. The premise of this algorithm is to get a short list of people you should talk to from your manager, ask them each for 30 minutes of their time, then do the following:
For the first 25 minutes: ask them to tell you everything they think you should know. Take copious notes. Only stop them to ask about things you don’t understand. Always stop them to ask about things you don’t understand.
For the next 3 minutes: ask about the biggest challenges the team has right now.
In the final 2 minutes: ask who else you should talk to. Write down every name they give you.
Repeat the above process for every name you’re given. Don’t stop until there are no new names.
Setting up tools
This one is a bit more straightforward, but your new data scientist will need some time to get their environment and tools all set up. This normally means jumping through more than a few hoops or requesting access for various accounts and software applications.
Anything you can do to remove friction in this process should be done. We have all had trouble running certain software or packages before. We know how frustrating it is. Sharing appropriate documentation, shortening the feedback loop between employee and service desk, and specifying team members to contact with questions are all good moves here.
Know the customer
No matter what industry and business model you are working with, data scientists need to know their customer. Domain knowledge is absolutely essential to consistently drive impact. A strong initial approach to developing this domain knowledge is learning about your customer segments and the demographics that they fall in.
“We think of data as the voice of our users at scale.” — Elena Grewal
Even better, the new hire should get their hands dirty and use the product if possible. You’ll find that the simple act of being your own customer can open up doors that you didn’t know existed when generating hypotheses and deriving insights from the data later on.
Quick history lesson
We know that history is bound to repeat itself, more than we care to admit sometimes. That’s why understanding past projects, initiatives, and insights within both the team and company can make a data scientist’s life easier.
They should take the time to review any past analysis or projects that are relevant to the team. Even skimming through some stuff will give new hires a feel for what’s been done before, allowing them to follow-up with someone if they are working on similar projects. They shouldn’t get stuck or waste time reinventing the wheel.
Perhaps more importantly, make sure there’s a clear understanding of the company’s vision and road map, along with the reasons they are doing the things that they’re doing.
Data scientists are thinkers. We have more time to ponder problems than PMs or engineers, so a solid understanding of the big picture can go a long, long way when it comes to making an impact.
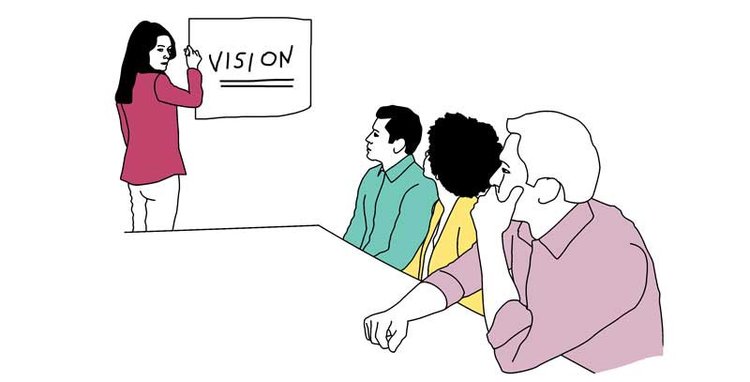
The first project
As soon as your data scientist has their environment set up, have them get started with an initial project. Ideally, you’ve reduced the technical and company-wide onboarding enough to get this project going within the first week or two.
This can be something small and concrete like a follow-up analysis or a one-off inquiry from a stakeholder. It’s okay if it doesn’t produce earth-shattering results. The goal of this project is to get the employee’s feet wet and jump-start them into doing more impactful work.
Once they have made some progress here, it’s a good idea to give them something more exploratory to dive into as well. Let them flex their creative muscles a bit and explore the data to try and solve a vague problem. This flexibility will go a long way in developing their comfortability with both the data and their new workflow.
Just-in-time vs. just-in-case
The reality is that nobody can get fully up to speed right away. It’s going to be a process where prioritizing the most important areas are key. Because of this, it’s important to emphasize that new data scientists focus their precious time on just-in-time skills and tools during their onboarding, rather than spreading themselves too thin with just-in-case things.
More concretely, an example of just-in-time learning would be getting familiar with your company’s data warehouse of choice, something that they’ll need to know early on. On the other hand, studying survival analysis because it could be applicable isn’t a priority during the onboarding process, and therefore fits into the just-in-case grouping.
This isn’t always going to be the case — there should be a balance. But it’s a good way to kick start things more quickly.
Documentation and feedback
The importance of documentation in this process can’t be overstated. Having a centralized location of the knowledge necessary for getting started can make onboarding run exponentially smoother. Building this out the right way may take some time up front, but it’ll pay itself off in the long-term.
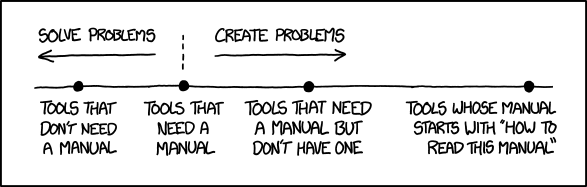
Lastly, collect feedback on the process and improve it for the next addition to the team. It’s difficult to put yourself in the shoes of someone that’s just starting out, so don’t depend on it. Gather feedback and poke at some of the potential weaknesses in the process so that you can brainstorm and eventually implement potential solutions. Your future hires will thank you.
Thanks for reading! If you enjoyed this post and you’re feeling generous, perhaps follow me on Twitter. You can also subscribe in the form below to get future posts like this one straight to your inbox. 🔥